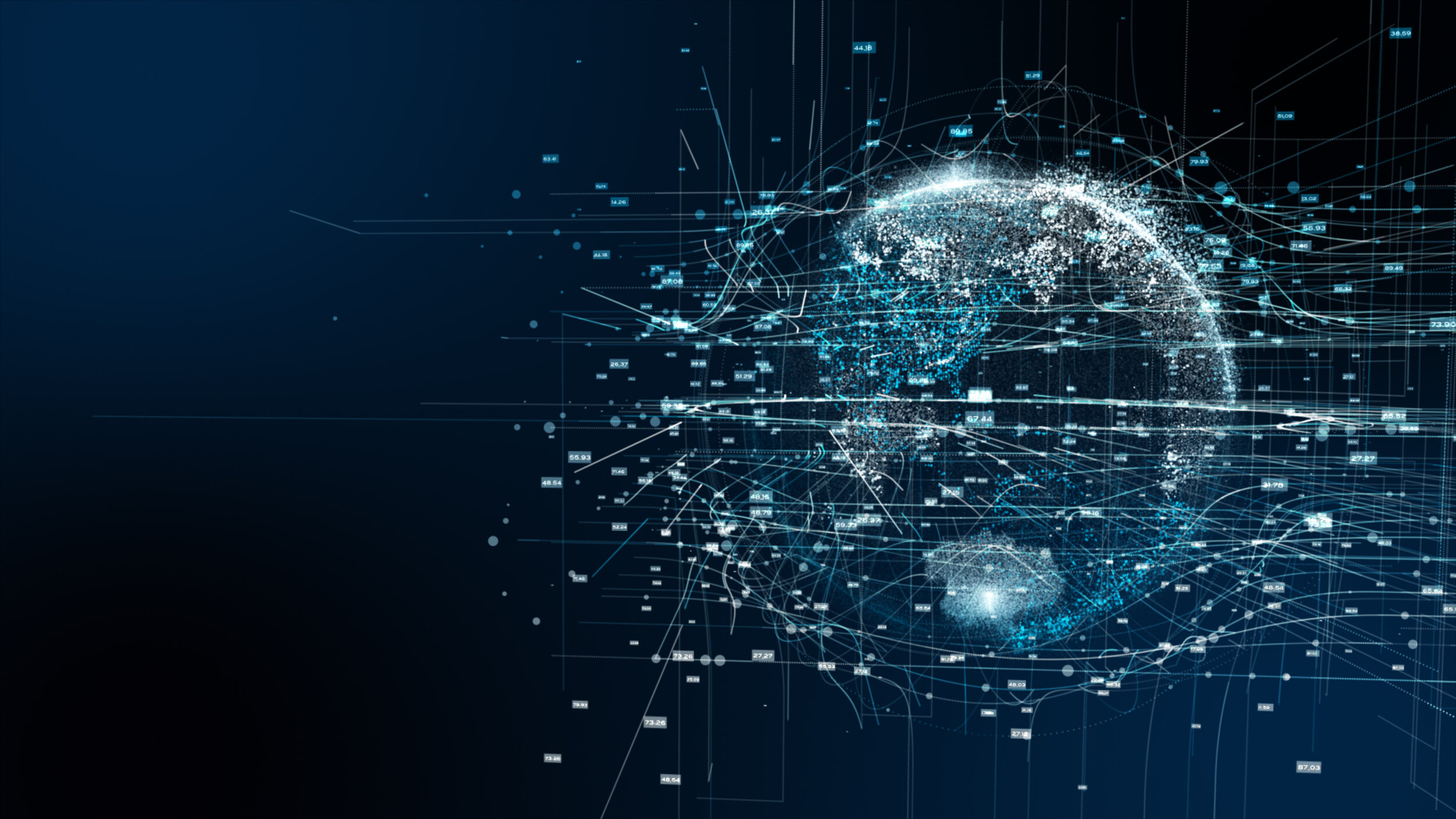
“To understand complex technological systems requires an understanding of how they are designed, how their realities are constructed within the possibilities created by their designers.”
– Klaus Krippendorff
AI and the Law
State Bars Beware - AI Chatbots and Unlicensed Practice of Law
on LinkedIn
State Bar Associations need to begin to consider how they will address the practice of law through AI technology
Machine Learning, Bias and the Law
on LinkedIn
When we lawyers look at technology, it's often from the perspective of what the technology can do for our field. But it may actually be more important to consider what technology is doing to our field.
Platform Companies Beware —
Proposed EU AI Regulations and Risk as a Competitive Advantage
in Data Driven Investor
There is an abiding belief in Silicon Valley that the enablers of AI technology bear no responsibility for how it is used when it is released into the world. This shield was essentially dismantled by the EU’s Proposed Artificial Intelligence Act.
Part 1
The issues we're seeing with AI applications aren't the result of poor design, bad intent or negligence. It's a communication problem. These issues require a soft-skill fix.
* Introduction to the AI Development Communication Cycle.
Part 2
The world isn't generic. The AI we create can't be either. Each party has a role in ensuring that we create ethical AI that is built with the end goal in mind. Product managers are uniquely suited to broker those initial conversations.
* Customer - PM Communication
Part 3
An AI system that is designed without a solid vision of its purpose will fail. If the design is to be successful, the vision must be accurately relayed from the customer to the developer by the product manager.
* PM - Developer Communication
Technical Best Practices - Product Management Chapter
by Foundation for Best Practices in Machine Learning
There is an oft-cited statistic that indicates that a staggering percentage of machine learning projects fail. A
variety of reasons are given for these failures, but the most cited reasons are in areas that are under the purview
of product management e.g. inadequate budget, unreasonable stakeholder expectations, unrealistic time frame,
poor market fit, biased or unfair outcomes, inadequate business value, insufficient internal infrastructure, lack of
communication and alignment between stakeholders, etc.
While the Product Manager, or the Product Management team, may not be directly responsible for each of these areas of competency, it is the role of Product Management to broker the relationships necessary to ensure all relevant issues to the product are identified and addressed and that information flows freely and as necessary to relevant stakeholders. The Best Practices attempt to highlight the various areas, analyses and decision points have been acknowledged within the industry as critical to the management of machine learning products.
Ethics and Natural Language Processing
Ethics in NLP - Helping customers determine if what we created is fit for their purposes
on LinkedIn
The purpose of NLP/NLU is to teach machines to talk to like humans. But how do humans talk? The assumption is that the data we use for training and the models we train it with are universally applicable. But clearly all humans don't speak the same language. And even when we technically do speak the same language, we speak it in a myriad of different ways. So how can we be building conversational AI, when we aren't training it with data that captures the way most of us actually converse?